Smart Manufacturing and Industry 4.0
Smart Manufacturing and Industry 4.0 represent the convergence of digital technologies and advanced manufacturing practices to create highly connected, efficient, and intelligent production environments. By integrating the Internet of Things (IoT), sensors, data analytics, artificial intelligence (AI), and robotics, these systems enable real-time monitoring, predictive decision-making, and greater adaptability to dynamic market demands. Often referred to as the “Fourth Industrial Revolution,” Industry 4.0 transforms traditional manufacturing into a digital ecosystem that optimizes productivity, reduces waste, and enhances flexibility.
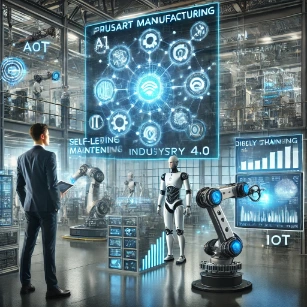
Table of Contents
Core Principles of Smart Manufacturing and Industry 4.0
Interconnectivity
- Definition:
- Devices, machines, and systems are connected through IoT to communicate and exchange data seamlessly.
- Applications:
- Smart sensors monitor equipment performance and send data to cloud-based systems.
Real-Time Data Collection and Analysis
- Definition:
- Sensors and connected devices collect real-time data from manufacturing operations.
- Applications:
- Analyzing production metrics to optimize workflows and reduce downtime.
Decentralized Decision-Making
- Definition:
- Machines and systems make decisions independently based on real-time data.
- Applications:
- Autonomous robots rerouting workflows when detecting bottlenecks.
Cyber-Physical Systems (CPS)
- Definition:
- Integration of physical processes with computational models to enable intelligent control and coordination.
- Applications:
- Self-regulating systems that adjust production parameters based on performance metrics.
Advanced Automation
- Definition:
- Robotics, AI, and machine learning automate repetitive or complex tasks.
- Applications:
- Robotic arms assembling intricate components in high-tech industries.
Digital Twins
- Definition:
- Virtual replicas of physical systems used for simulation, monitoring, and optimization.
- Applications:
- Testing changes in production processes virtually before implementation.
Key Technologies Driving Smart Manufacturing and Industry 4.0
Internet of Things (IoT)
- Role:
- Connects devices, sensors, and machines for seamless data communication.
- Applications:
- Real-time monitoring of production lines, equipment health, and inventory levels.
Big Data and Advanced Analytics
- Role:
- Processes vast amounts of data to uncover patterns and trends.
- Applications:
- Predicting machine failures using historical performance data.
Artificial Intelligence (AI) and Machine Learning
- Role:
- Enables predictive maintenance, demand forecasting, and quality control.
- Applications:
- AI systems detecting anomalies in product quality during production.
Robotics and Automation
- Role:
- Replaces or enhances manual labor with automated systems.
- Applications:
- Collaborative robots (cobots) working alongside humans in assembly lines.
Cloud Computing
- Role:
- Provides scalable storage and processing power for smart manufacturing systems.
- Applications:
- Cloud-based platforms for global supply chain integration and monitoring.
Blockchain
- Role:
- Ensures transparency and security in supply chains.
- Applications:
- Verifying the authenticity of raw materials and tracking their origin.
Augmented Reality (AR) and Virtual Reality (VR)
- Role:
- Enhances training, maintenance, and design visualization.
- Applications:
- AR guides workers in assembly tasks by overlaying instructions on physical components.
Edge Computing
- Role:
- Processes data locally at the source to reduce latency and bandwidth usage.
- Applications:
- Real-time control of manufacturing equipment with minimal delay.
5G Connectivity
- Role:
- Provides ultra-fast and low-latency communication for IoT devices.
- Applications:
- Enabling seamless communication between smart machines and systems.
Applications of Smart Manufacturing and Industry 4.0
Predictive Maintenance
- Definition:
- Using sensors and AI to predict equipment failures before they occur.
- Benefits:
- Reduces downtime and maintenance costs.
- Example:
- Sensors monitoring vibrations in machinery to predict bearing failures.
Quality Control
- Definition:
- Real-time inspection of products using AI and machine vision systems.
- Benefits:
- Ensures consistent product quality and reduces defects.
- Example:
- Cameras inspecting electronics for soldering defects.
Flexible Manufacturing Systems
- Definition:
- Systems capable of adapting to changes in product design or volume without major reconfiguration.
- Benefits:
- Shortens production cycles and enhances responsiveness to market changes.
- Example:
- Automotive plants switching between different car models on the same production line.
Supply Chain Optimization
- Definition:
- Real-time tracking and analysis of supply chain data.
- Benefits:
- Improves inventory management and reduces logistics costs.
- Example:
- IoT-enabled systems tracking shipment locations and conditions.
Energy Management
- Definition:
- Monitoring and optimizing energy consumption across manufacturing facilities.
- Benefits:
- Reduces operational costs and environmental impact.
- Example:
- Smart grids adjusting energy usage based on real-time demand.
Human-Machine Collaboration
- Definition:
- Integration of collaborative robots (cobots) to assist workers.
- Benefits:
- Enhances worker safety and productivity.
- Example:
- Cobots lifting heavy components while workers handle precision assembly.
Mass Customization
- Definition:
- Producing highly personalized products at scale.
- Benefits:
- Increases customer satisfaction and competitiveness.
- Example:
- 3D printing personalized medical devices like prosthetics.
Benefits of Smart Manufacturing and Industry 4.0
- Increased Productivity:
- Automation and real-time optimization reduce inefficiencies.
- Enhanced Flexibility:
- Quickly adapts to changes in demand or production requirements.
- Improved Quality:
- AI-driven systems detect and correct defects in real-time.
- Cost Savings:
- Reduces material waste, energy consumption, and downtime.
- Sustainability:
- Optimizes resource usage and reduces environmental impact.
- Enhanced Decision-Making:
- Real-time data enables informed and swift decisions.
Challenges in Implementing Smart Manufacturing and Industry 4.0
- High Initial Costs:
- Significant investment required for infrastructure, hardware, and training.
- Cybersecurity Risks:
- Increased connectivity exposes systems to potential cyberattacks.
- Data Management:
- Handling and analyzing vast amounts of data from IoT devices.
- Workforce Transition:
- Reskilling employees to work with advanced technologies.
- Interoperability Issues:
- Ensuring compatibility between legacy systems and new technologies.
Emerging Trends in Smart Manufacturing and Industry 4.0
Autonomous Manufacturing
- Fully autonomous production systems capable of self-optimization and adaptation.
- Example: Lights-out manufacturing facilities.
Green Manufacturing
- Integrating sustainable practices into smart factories to reduce carbon footprints.
- Example: Using renewable energy sources and recycling waste materials.
AI-Driven Optimization
- AI models analyzing historical and real-time data to optimize processes and predict trends.
- Example: AI adjusting production schedules based on demand forecasts.
Digital Thread
- A continuous flow of data across the product lifecycle, from design to disposal.
- Example: Tracking product performance post-sale for feedback into design improvements.
Additive Manufacturing Integration
- Combining 3D printing with smart systems for customized and efficient production.
- Example: On-demand production of spare parts.
Future Directions for Smart Manufacturing and Industry 4.0
- Hyperconnectivity:
- Expanded use of IoT devices to connect every aspect of production.
- Collaborative Ecosystems:
- Manufacturers, suppliers, and distributors working in integrated networks.
- Artificial Intelligence Dominance:
- AI driving end-to-end automation and decision-making.
- Quantum Computing:
- Enhancing complex simulations and optimizations in manufacturing.
- Edge-to-Cloud Integration:
- Combining edge computing for real-time operations with cloud computing for large-scale analytics.
Smart Manufacturing and Industry 4.0: Conclusion
Smart Manufacturing and Industry 4.0 are revolutionizing the manufacturing landscape by creating intelligent, agile, and efficient production environments. Through advanced technologies like IoT, AI, robotics, and digital twins, these systems enable real-time monitoring, predictive decision-making, and enhanced collaboration. As industries increasingly adopt these practices, smart manufacturing will play a central role in driving innovation, sustainability, and global competitiveness in the years to come.
Smart Manufacturing and Industry 4.0: Review Questions with Detailed Answers
1. What is Industry 4.0, and how does it revolutionize modern manufacturing?
Answer:
Industry 4.0, also known as the Fourth Industrial Revolution, refers to the integration of digital technologies into manufacturing processes. It encompasses advancements such as the Internet of Things (IoT), artificial intelligence (AI), robotics, big data analytics, and cloud computing. Industry 4.0 revolutionizes modern manufacturing by enabling smarter, more efficient, and highly automated production systems. Key benefits include increased productivity, enhanced flexibility, improved quality, reduced operational costs, and the ability to quickly adapt to changing market demands. By leveraging real-time data and interconnected systems, Industry 4.0 facilitates predictive maintenance, optimized supply chains, and personalized manufacturing, thereby transforming the traditional manufacturing landscape into a more agile and intelligent ecosystem.
2. How do IoT and connected devices contribute to Smart Manufacturing under Industry 4.0?
Answer:
The Internet of Things (IoT) and connected devices are fundamental components of Smart Manufacturing within the Industry 4.0 framework. IoT enables the seamless connectivity of machines, sensors, and devices across the manufacturing floor, allowing for real-time data collection and communication. This connectivity facilitates several key contributions:
Real-Time Monitoring: Sensors on machinery track performance metrics such as temperature, vibration, and speed, enabling continuous monitoring of equipment health and process conditions.
Predictive Maintenance: By analyzing data from connected devices, manufacturers can predict when equipment is likely to fail, allowing for timely maintenance and reducing unplanned downtime.
Enhanced Efficiency: IoT devices optimize production workflows by providing accurate data on resource utilization, enabling better scheduling, and minimizing waste.
Supply Chain Integration: Connected devices facilitate end-to-end visibility across the supply chain, improving coordination with suppliers, reducing lead times, and enhancing inventory management.
Data-Driven Decision Making: The vast amount of data generated by IoT devices is analyzed using big data analytics and AI, providing insights that drive strategic decisions and continuous improvement.
Overall, IoT and connected devices empower manufacturers to create more responsive, efficient, and intelligent production environments, significantly enhancing the capabilities and competitiveness of manufacturing operations under Industry 4.0.
3. What role does artificial intelligence (AI) play in optimizing manufacturing processes in Industry 4.0?
Answer:
Artificial Intelligence (AI) plays a pivotal role in optimizing manufacturing processes within the Industry 4.0 paradigm by enabling smarter, more adaptive, and highly efficient operations. Key roles of AI in manufacturing include:
Predictive Maintenance: AI algorithms analyze data from machinery sensors to predict potential failures before they occur, allowing for proactive maintenance and reducing downtime.
Quality Control: AI-powered computer vision systems inspect products in real-time, identifying defects with higher accuracy and speed than manual inspections, thus ensuring consistent product quality.
Supply Chain Optimization: AI enhances supply chain management by forecasting demand, optimizing inventory levels, and improving logistics, resulting in reduced costs and increased responsiveness.
Process Automation: AI-driven robots and automated systems handle complex tasks with precision, increasing production speed and reducing human error.
Energy Management: AI optimizes energy usage by analyzing consumption patterns and adjusting processes to minimize energy waste, leading to cost savings and sustainability.
Customization and Flexibility: AI enables mass customization by quickly adapting production processes to meet specific customer requirements without sacrificing efficiency.
Data Analytics and Insights: AI analyzes vast amounts of data to uncover patterns and insights that inform strategic decisions, driving continuous improvement and innovation in manufacturing processes.
By integrating AI into manufacturing, Industry 4.0 fosters a more intelligent and efficient production environment, enhancing overall operational performance, reducing costs, and enabling manufacturers to remain competitive in a rapidly evolving market.
4. How does additive manufacturing (3D printing) fit into the framework of Industry 4.0?
Answer:
Additive manufacturing, commonly known as 3D printing, is a key component of Industry 4.0, integrating seamlessly into the digital and automated manufacturing landscape. Its role within Industry 4.0 includes:
Customization and Flexibility: Additive manufacturing allows for the production of highly customized products without the need for expensive tooling, enabling manufacturers to meet specific customer requirements and adapt quickly to changing demands.
Rapid Prototyping: It facilitates the quick creation of prototypes, reducing the time and cost associated with product development cycles and accelerating innovation.
Complex Geometries: 3D printing enables the production of complex and lightweight structures that are difficult or impossible to achieve with traditional manufacturing methods, enhancing product performance and material efficiency.
On-Demand Production: Additive manufacturing supports on-demand production, reducing the need for large inventories and allowing for just-in-time manufacturing practices.
Integration with Digital Systems: It works seamlessly with digital design and simulation tools, allowing for real-time adjustments and optimizations in the manufacturing process.
Sustainability: Additive manufacturing minimizes material waste by building products layer by layer, improving resource utilization and reducing the environmental impact of production.
Supply Chain Optimization: By enabling decentralized production, additive manufacturing reduces dependence on centralized manufacturing facilities and long supply chains, enhancing resilience and reducing lead times.
In the Industry 4.0 framework, additive manufacturing complements other technologies such as IoT, AI, and robotics, contributing to a more integrated, flexible, and efficient manufacturing ecosystem. It empowers manufacturers to innovate, respond swiftly to market changes, and produce high-quality, customized products with greater efficiency.
5. What is the significance of data analytics in Smart Manufacturing and Industry 4.0?
Answer:
Data analytics is a cornerstone of Smart Manufacturing and Industry 4.0, playing a crucial role in transforming raw data into actionable insights that drive informed decision-making and continuous improvement. The significance of data analytics in this context includes:
Enhanced Decision-Making:
- Significance: Data analytics provides real-time insights into manufacturing operations, enabling managers to make informed decisions based on accurate and timely information.
- Impact: Improves operational efficiency, optimizes resource allocation, and enhances strategic planning.
Predictive Maintenance:
- Significance: Analyzing data from machinery sensors helps predict potential equipment failures before they occur.
- Impact: Reduces unplanned downtime, extends the lifespan of equipment, and lowers maintenance costs by enabling proactive maintenance scheduling.
Process Optimization:
- Significance: Data analytics identifies inefficiencies and bottlenecks in manufacturing processes.
- Impact: Enables targeted improvements that enhance productivity, reduce cycle times, and minimize waste.
Quality Improvement:
- Significance: Analyzing quality-related data helps identify trends and root causes of defects.
- Impact: Facilitates continuous quality enhancement, reducing defect rates, and ensuring consistent product quality.
Supply Chain Management:
- Significance: Data analytics enhances visibility and coordination across the supply chain.
- Impact: Improves inventory management, optimizes logistics, and ensures timely delivery of materials and products.
Energy Efficiency:
- Significance: Monitoring and analyzing energy consumption data helps identify opportunities for energy savings.
- Impact: Reduces operational costs and supports sustainability initiatives by minimizing energy waste.
Customization and Personalization:
- Significance: Data analytics enables the analysis of customer preferences and market trends.
- Impact: Supports mass customization and personalized manufacturing, meeting specific customer demands more effectively.
Risk Management:
- Significance: Data analytics assesses and mitigates risks by identifying potential disruptions and vulnerabilities in manufacturing processes.
- Impact: Enhances operational resilience and ensures smoother production continuity.
Innovation and Development:
- Significance: Analyzing data from various sources fosters innovation by uncovering new opportunities and areas for development.
- Impact: Drives the creation of new products, services, and manufacturing techniques, keeping the organization competitive.
Compliance and Reporting:
- Significance: Data analytics ensures that manufacturing processes comply with industry standards and regulatory requirements.
- Impact: Simplifies reporting, reduces the risk of non-compliance penalties, and enhances the organization’s credibility.
Benefits of Big Data and Analytics in Industry 4.0:
- Improved Efficiency: Streamlines operations by identifying and eliminating inefficiencies.
- Enhanced Quality: Ensures consistent product quality through continuous monitoring and improvement.
- Cost Savings: Reduces costs associated with defects, maintenance, and energy consumption.
- Innovation: Drives innovation by uncovering new opportunities and insights from data.
- Agility: Enables manufacturers to quickly adapt to changing market conditions and customer demands.
- Competitive Advantage: Provides a data-driven approach that enhances the organization’s ability to compete effectively in the market.
Case Example: A manufacturing company implemented a big data analytics platform to analyze data from its production lines. By leveraging predictive analytics, they identified patterns indicating early signs of equipment wear. This allowed them to schedule maintenance before failures occurred, reducing downtime by 40% and saving significant maintenance costs. Additionally, process optimization based on data insights led to a 25% increase in production efficiency and improved product quality, resulting in higher customer satisfaction and increased sales.
Conclusion: Data analytics is integral to the success of Smart Manufacturing and Industry 4.0, providing the foundation for intelligent and efficient manufacturing operations. By leveraging data-driven insights, manufacturers can optimize processes, improve quality, reduce costs, enhance customer satisfaction, and drive continuous innovation, ultimately achieving a competitive advantage in the market.
6. How do robotics and automation technologies enhance production efficiency in Industry 4.0?
Answer:
Robotics and automation technologies are fundamental enablers of Industry 4.0, significantly enhancing production efficiency through increased speed, precision, and reliability. Their integration into manufacturing processes brings several key benefits:
Increased Productivity:
- Enhancement: Robots and automated systems can operate continuously without fatigue, leading to higher production rates compared to manual labor.
- Impact: Increases overall output and meets growing demand more effectively.
Precision and Consistency:
- Enhancement: Automation ensures high levels of precision and repeatability in manufacturing processes.
- Impact: Reduces defects and variability, leading to superior product quality and reliability.
Cost Reduction:
- Enhancement: Automation minimizes labor costs and reduces the likelihood of human errors.
- Impact: Lowers overall production costs and enhances profitability.
Safety Improvement:
- Enhancement: Robots can handle hazardous tasks, such as working with toxic materials or performing heavy lifting.
- Impact: Reduces workplace accidents and improves overall safety conditions for human workers.
Flexibility and Scalability:
- Enhancement: Modern robotic systems are programmable and adaptable to different tasks and product types.
- Impact: Facilitates quick adjustments to production lines and scalability to meet changing market demands.
Data Integration and Analytics:
- Enhancement: Automated systems can collect and transmit data on performance metrics in real-time.
- Impact: Supports data-driven decision-making, process optimization, and continuous improvement initiatives.
Resource Optimization:
- Enhancement: Automation optimizes the use of materials and energy through precise control of manufacturing processes.
- Impact: Reduces waste, lowers energy consumption, and promotes sustainable manufacturing practices.
Enhanced Supply Chain Coordination:
- Enhancement: Automation integrates seamlessly with supply chain systems, improving synchronization and flow of materials.
- Impact: Enhances overall supply chain efficiency, reducing lead times and ensuring timely production.
Rapid Prototyping and Innovation:
- Enhancement: Automation facilitates the rapid production of prototypes and small batches.
- Impact: Accelerates product development cycles and supports innovation by enabling quick iterations and testing.
Employee Augmentation:
- Enhancement: Robots and automated systems can complement human workers, handling repetitive or strenuous tasks while humans focus on more complex and creative activities.
- Impact: Enhances workforce efficiency and job satisfaction by allowing employees to engage in higher-value tasks.
Case Example: A car manufacturer integrated automated robotic arms into its assembly line to handle welding and painting tasks. This integration resulted in a 30% increase in production speed, a 25% reduction in defects, and a 20% decrease in operational costs. Additionally, the automation improved workplace safety by minimizing human exposure to hazardous processes, demonstrating the significant impact of robotics and automation on production efficiency.
Conclusion: Robotics and automation technologies are essential components of Industry 4.0, driving substantial improvements in production efficiency, product quality, and operational costs. By leveraging these technologies, manufacturers can achieve higher productivity, enhanced safety, greater flexibility, and sustained competitive advantage in a rapidly evolving industrial landscape.
7. What are the key challenges faced by manufacturers in implementing Smart Manufacturing and Industry 4.0 technologies, and how can they be addressed?
Answer:
Implementing Smart Manufacturing and Industry 4.0 technologies presents several challenges for manufacturers. Addressing these challenges is essential for successful integration and realization of the benefits associated with Industry 4.0. The key challenges and potential solutions include:
High Initial Investment Costs:
- Challenge: Significant capital investment is required for acquiring advanced technologies, upgrading infrastructure, and training employees.
- Solution: Manufacturers can adopt a phased implementation approach, starting with pilot projects to demonstrate value and secure funding. Leveraging government grants, incentives, and financing options can also mitigate financial burdens.
Cybersecurity Risks:
- Challenge: Increased connectivity and data exchange heighten the risk of cyberattacks and data breaches.
- Solution: Implement robust cybersecurity measures, including firewalls, encryption, access controls, and regular security audits. Training employees on cybersecurity best practices and developing incident response plans are also crucial.
Integration with Legacy Systems:
- Challenge: Integrating new Industry 4.0 technologies with existing legacy systems can be complex and resource-intensive.
- Solution: Use middleware and integration platforms to facilitate seamless communication between old and new systems. Additionally, conducting thorough compatibility assessments and planning for system upgrades can ease integration challenges.
Skill Gaps and Workforce Readiness:
- Challenge: The adoption of advanced technologies requires new skill sets that may be lacking in the current workforce.
- Solution: Invest in comprehensive training and development programs to upskill employees. Partnering with educational institutions and providing continuous learning opportunities can bridge skill gaps.
Data Management and Analysis:
- Challenge: Handling the vast amount of data generated by smart manufacturing technologies can overwhelm existing data management systems.
- Solution: Implement advanced data management platforms and utilize big data analytics tools to efficiently store, process, and analyze data. Establishing clear data governance policies ensures data quality and accessibility.
Change Management and Organizational Culture:
- Challenge: Resistance to change and a lack of supportive organizational culture can impede the adoption of new technologies.
- Solution: Foster a culture of innovation and continuous improvement by involving employees in the transition process. Communicate the benefits of Industry 4.0, provide incentives for adoption, and ensure leadership support to drive change.
Interoperability and Standardization:
- Challenge: Lack of standardized protocols and interoperability between different technologies and devices can hinder integration efforts.
- Solution: Adopt industry standards and protocols to ensure compatibility and interoperability. Participating in industry consortia and collaborating with technology providers can also promote standardization.
Scalability and Flexibility:
- Challenge: Ensuring that smart manufacturing technologies can scale with business growth and adapt to changing requirements is essential.
- Solution: Choose scalable and flexible technologies that can grow with the organization. Modular and cloud-based solutions offer the adaptability needed to accommodate future expansions and evolving needs.
Return on Investment (ROI) Uncertainty:
- Challenge: Unclear or uncertain ROI can make it difficult to justify the investment in smart manufacturing technologies.
- Solution: Conduct thorough ROI analyses and establish clear metrics for measuring success. Piloting technologies and gathering data on performance improvements can help demonstrate value and secure stakeholder buy-in.
Supply Chain Complexity:
- Challenge: Integrating smart manufacturing with complex and global supply chains can complicate operations and coordination.
- Solution: Enhance supply chain visibility through IoT and data analytics, improve collaboration with suppliers, and adopt flexible supply chain strategies to manage complexity effectively.
Conclusion: Manufacturers face numerous challenges in implementing Smart Manufacturing and Industry 4.0 technologies, including financial, technical, and organizational obstacles. By adopting strategic approaches such as phased implementation, robust cybersecurity measures, comprehensive training programs, and fostering a culture of continuous improvement, manufacturers can overcome these challenges and successfully integrate Industry 4.0 technologies. Addressing these challenges effectively enables organizations to realize the full potential of Smart Manufacturing, driving efficiency, innovation, and competitive advantage in the modern industrial landscape.
8. What is the role of Big Data and analytics in Industry 4.0, and how do they impact decision-making in manufacturing?
Answer:
Big Data and analytics are integral to Industry 4.0, playing a crucial role in transforming raw data into valuable insights that enhance decision-making in manufacturing. Their role and impact include:
Data Collection and Integration:
- Role: Big Data encompasses the vast volumes of structured and unstructured data generated by various sources, including IoT sensors, machines, production systems, and supply chain activities.
- Impact: Enables comprehensive data collection and integration, providing a holistic view of manufacturing operations.
Real-Time Monitoring and Control:
- Role: Analytics tools process and analyze real-time data to monitor production processes continuously.
- Impact: Allows for immediate detection of anomalies, enabling prompt corrective actions to maintain process stability and quality.
Predictive Maintenance:
- Role: Analyzing historical and real-time data to predict equipment failures before they occur.
- Impact: Reduces unplanned downtime, extends the lifespan of machinery, and lowers maintenance costs by enabling proactive maintenance scheduling.
Process Optimization:
- Role: Utilizing data analytics to identify inefficiencies and optimize manufacturing processes.
- Impact: Enhances operational efficiency, reduces cycle times, minimizes waste, and improves overall productivity.
Quality Assurance and Control:
- Role: Analyzing quality data to identify patterns, trends, and root causes of defects.
- Impact: Facilitates continuous quality improvement, reduces defect rates, and ensures consistent product quality.
Supply Chain Optimization:
- Role: Leveraging data analytics to enhance supply chain visibility, forecast demand, and optimize inventory levels.
- Impact: Improves supply chain efficiency, reduces lead times, and ensures timely availability of materials, supporting Just-In-Time (JIT) production.
Customer Insights and Personalization:
- Role: Analyzing customer data and market trends to understand preferences and demand patterns.
- Impact: Enables manufacturers to tailor products and services to meet specific customer needs, enhancing customer satisfaction and loyalty.
Energy Management:
- Role: Monitoring and analyzing energy consumption data to identify opportunities for energy savings.
- Impact: Reduces energy costs, supports sustainability initiatives, and minimizes the environmental impact of manufacturing operations.
Strategic Decision-Making:
- Role: Providing data-driven insights that inform strategic business decisions related to production planning, resource allocation, and market expansion.
- Impact: Enhances the accuracy and effectiveness of decision-making, leading to better business outcomes and competitive advantage.
Risk Management:
- Role: Identifying potential risks and vulnerabilities through data analysis.
- Impact: Enables proactive risk mitigation strategies, ensuring smoother and more resilient manufacturing operations.
Benefits of Big Data and Analytics in Industry 4.0:
- Improved Efficiency: Streamlines operations by identifying and eliminating inefficiencies.
- Enhanced Quality: Ensures consistent product quality through continuous monitoring and improvement.
- Cost Savings: Reduces costs associated with defects, maintenance, and energy consumption.
- Innovation: Drives innovation by uncovering new opportunities and insights from data.
- Agility: Enables manufacturers to quickly adapt to changing market conditions and customer demands.
- Competitive Advantage: Provides a data-driven approach that enhances the organization’s ability to compete effectively in the market.
Case Example: A manufacturing company implemented a big data analytics platform to analyze data from its production lines. By leveraging predictive analytics, they identified patterns indicating early signs of equipment wear. This allowed them to schedule maintenance before failures occurred, reducing downtime by 40% and saving significant maintenance costs. Additionally, process optimization based on data insights led to a 25% increase in production efficiency and improved product quality, resulting in higher customer satisfaction and increased sales.
Conclusion: Big Data and analytics are transformative forces in Industry 4.0, enabling manufacturers to harness vast amounts of data for informed decision-making, process optimization, and continuous improvement. By integrating big data analytics into their operations, manufacturers can enhance efficiency, quality, and competitiveness, ultimately achieving a competitive advantage in the market.
9. How do collaborative robots (cobots) differ from traditional industrial robots, and what advantages do they offer in Smart Manufacturing?
Answer:
Collaborative robots, commonly known as cobots, differ from traditional industrial robots in several key aspects, including their design, functionality, interaction capabilities, and intended use within manufacturing environments. The advantages they offer in Smart Manufacturing are substantial, enhancing flexibility, safety, and efficiency.
Differences Between Cobots and Traditional Industrial Robots:
Aspect | Collaborative Robots (Cobots) | Traditional Industrial Robots |
---|---|---|
Design and Size | Smaller, lightweight, and often more flexible | Larger and more rigid, designed for heavy-duty tasks |
Operation Mode | Designed to work alongside humans safely | Typically operate in segregated, fenced-off areas |
Safety Features | Equipped with sensors and force-limiting technology to ensure safe human-robot interaction | Require safety cages and barriers to prevent human contact |
Programming and Use | Easy to program and reconfigure for various tasks | Require specialized programming and are often task-specific |
Flexibility | Highly adaptable and capable of handling multiple tasks | Primarily designed for repetitive, fixed tasks |
Cost | Generally more affordable and accessible for small to medium-sized enterprises (SMEs) | Higher initial investment, often suited for large-scale operations |
Integration | Easily integrated into existing workflows without extensive modifications | Often require significant changes to production lines for integration |
Learning Capability | Often equipped with AI and machine learning for improved adaptability | Limited adaptability and learning capabilities |
Advantages of Cobots in Smart Manufacturing:
Enhanced Flexibility:
- Advantage: Cobots can be easily reprogrammed and redeployed for different tasks, allowing manufacturers to adapt quickly to changing production needs.
- Impact: Increases operational agility and supports diverse manufacturing processes without significant downtime.
Improved Safety:
- Advantage: Cobots are designed to work safely alongside human workers, with features such as force sensing and collision detection.
- Impact: Reduces the risk of workplace injuries, enhances worker safety, and promotes a collaborative working environment.
Cost-Effectiveness:
- Advantage: Cobots are generally more affordable and require less infrastructure investment compared to traditional industrial robots.
- Impact: Makes automation accessible to small and medium-sized enterprises, enabling wider adoption of smart manufacturing practices.
Increased Productivity:
- Advantage: Cobots can handle repetitive and mundane tasks, allowing human workers to focus on more complex and value-added activities.
- Impact: Boosts overall productivity and efficiency by optimizing the division of labor between humans and robots.
Ease of Integration:
- Advantage: Cobots are designed to integrate seamlessly into existing production lines with minimal modifications.
- Impact: Facilitates smoother and faster implementation of automation technologies, reducing downtime and disruption.
Enhanced Precision and Consistency:
- Advantage: Cobots perform tasks with high precision and consistency, ensuring quality and reducing variability in production.
- Impact: Improves product quality, reduces defects, and enhances customer satisfaction.
Scalability:
- Advantage: Cobots can be easily scaled up or down based on production demands, allowing manufacturers to respond to market fluctuations.
- Impact: Provides the flexibility to expand or contract operations without significant capital investment.
Employee Satisfaction:
- Advantage: Cobots take over physically demanding and repetitive tasks, reducing worker fatigue and improving job satisfaction.
- Impact: Enhances employee morale and retention by allowing workers to engage in more fulfilling and creative tasks.
Case Example: A small electronics manufacturer implemented cobots to handle component assembly tasks. The cobots worked alongside human operators, performing repetitive and precise assembly steps. This integration led to a 30% increase in production speed, a 20% reduction in assembly errors, and improved worker satisfaction as employees could focus on quality control and innovation rather than repetitive tasks.
Conclusion: Collaborative robots offer significant advantages over traditional industrial robots, particularly in the context of Smart Manufacturing and Industry 4.0. Their flexibility, safety, cost-effectiveness, and ease of integration make them ideal for enhancing productivity and quality in modern manufacturing environments. By leveraging cobots, manufacturers can create more adaptable, efficient, and worker-friendly production systems, driving operational excellence and competitive advantage.
10. What are the environmental implications of adopting Smart Manufacturing and Industry 4.0 technologies, and how can they contribute to sustainability?
Answer:
Adopting Smart Manufacturing and Industry 4.0 technologies has significant environmental implications, offering numerous opportunities to enhance sustainability and reduce the ecological footprint of manufacturing operations. These technologies enable more efficient resource utilization, waste reduction, and energy conservation, contributing to greener and more sustainable manufacturing practices.
Environmental Implications and Contributions to Sustainability:
Energy Efficiency:
- Implication: Smart Manufacturing technologies such as IoT sensors and AI-driven energy management systems optimize energy usage.
- Contribution: Reduces overall energy consumption, lowers greenhouse gas emissions, and decreases operational costs through more efficient energy use.
Waste Reduction:
- Implication: Data analytics and process optimization tools identify and eliminate waste in production processes.
- Contribution: Minimizes material waste, reduces landfill usage, and conserves natural resources, leading to more sustainable manufacturing practices.
Resource Optimization:
- Implication: Technologies like predictive maintenance and just-in-time inventory management ensure optimal use of materials and resources.
- Contribution: Prevents overproduction, reduces excess inventory, and ensures efficient use of raw materials, minimizing resource depletion and environmental impact.
Sustainable Supply Chains:
- Implication: Enhanced visibility and coordination across supply chains through IoT and data analytics enable sustainable sourcing and logistics.
- Contribution: Promotes the use of environmentally friendly materials, reduces transportation emissions, and ensures compliance with sustainability standards.
Circular Economy Support:
- Implication: Smart Manufacturing facilitates product design for recyclability and reusability, supporting circular economy initiatives.
- Contribution: Enables the efficient recycling and repurposing of materials, reducing waste and promoting sustainable product lifecycles.
Emissions Monitoring and Control:
- Implication: Real-time monitoring systems track emissions and environmental impact throughout the manufacturing process.
- Contribution: Helps in adhering to environmental regulations, reducing pollutant emissions, and ensuring compliance with sustainability standards.
Reduced Carbon Footprint:
- Implication: Optimization of production processes and energy usage leads to a lower carbon footprint.
- Contribution: Contributes to global efforts to combat climate change by reducing the amount of greenhouse gases emitted by manufacturing activities.
Sustainable Product Development:
- Implication: AI and big data analytics support the design of eco-friendly products that require fewer resources and generate less waste.
- Contribution: Promotes the creation of sustainable products that are easier to manufacture, use, and dispose of responsibly.
Extended Equipment Lifespan:
- Implication: Predictive maintenance ensures that equipment is maintained in optimal condition, extending its operational lifespan.
- Contribution: Reduces the need for frequent replacements, thereby lowering material usage and waste generation.
Smart Building and Infrastructure:
- Implication: Integration of smart building technologies within manufacturing facilities optimizes energy usage for heating, cooling, and lighting.
- Contribution: Enhances overall energy efficiency of manufacturing facilities, leading to lower operational energy consumption and reduced environmental impact.
Benefits of Industry 4.0 Technologies for Sustainability:
- Enhanced Operational Efficiency: Streamlined processes and reduced resource consumption contribute to more sustainable manufacturing operations.
- Lower Environmental Impact: Reduced waste, emissions, and energy usage align with global sustainability goals and environmental regulations.
- Cost Savings: Efficient resource utilization and energy management lead to significant cost reductions, supporting both economic and environmental sustainability.
- Regulatory Compliance: Helps manufacturers meet stringent environmental regulations and standards, avoiding penalties and enhancing corporate responsibility.
- Brand Reputation: Demonstrates a commitment to sustainability, enhancing the organization’s reputation and attracting environmentally conscious customers and partners.
Case Example: A manufacturing company implemented an IoT-based energy management system to monitor and optimize energy usage across its production facilities. By analyzing real-time data, the company identified areas where energy consumption could be reduced and implemented automated controls to optimize lighting and machinery operation. This led to a 25% reduction in energy consumption, resulting in lower operational costs and a significant decrease in carbon emissions, thereby enhancing the company’s sustainability profile.
Conclusion: The adoption of Smart Manufacturing and Industry 4.0 technologies plays a vital role in advancing sustainability within the manufacturing sector. By leveraging advanced technologies to optimize resource usage, reduce waste and emissions, and enhance operational efficiency, manufacturers can significantly minimize their environmental impact. These technologies not only contribute to the achievement of environmental sustainability goals but also drive economic benefits and compliance with regulatory standards, positioning manufacturers for long-term success in a more sustainable and environmentally conscious market.
Conclusion:
The above review questions and detailed answers provide a comprehensive exploration of Smart Manufacturing and Industry 4.0. Covering essential topics such as Industry 4.0 fundamentals, IoT integration, AI optimization, additive manufacturing, data analytics, robotics and automation, implementation challenges, Big Data roles, collaborative robots, and environmental sustainability, these questions facilitate a deep understanding of how to leverage advanced technologies for smarter, more efficient, and sustainable manufacturing processes. Engaging with these questions equips students and professionals with the knowledge to implement best practices, overcome challenges, and achieve sustained excellence in the competitive landscape of modern manufacturin
Smart Manufacturing and Industry 4.0: Thought-Provoking Questions
1. What are the cybersecurity challenges associated with Industry 4.0, and how can manufacturers mitigate these risks?
Answer:
Cybersecurity Challenges in Industry 4.0:
Industry 4.0 introduces a highly interconnected and digitized manufacturing environment, which, while offering numerous benefits, also presents significant cybersecurity challenges:
Increased Attack Surface:
- Challenge: The integration of IoT devices, cloud computing, and interconnected systems expands the number of entry points for cyberattacks.
- Impact: Greater vulnerability to unauthorized access, data breaches, and malicious activities.
Legacy Systems Integration:
- Challenge: Integrating new Industry 4.0 technologies with older, legacy systems that may lack robust security features.
- Impact: Creates weak links that can be exploited by attackers, compromising the entire network.
Data Privacy and Protection:
- Challenge: Handling vast amounts of sensitive data, including intellectual property and personal information.
- Impact: Risk of data theft, manipulation, and non-compliance with data protection regulations.
Advanced Persistent Threats (APTs):
- Challenge: Sophisticated, long-term targeted attacks aimed at infiltrating and remaining undetected within manufacturing networks.
- Impact: Continuous disruption, espionage, and significant financial and reputational damage.
Lack of Cybersecurity Awareness:
- Challenge: Employees may lack adequate training and awareness regarding cybersecurity best practices.
- Impact: Increased susceptibility to phishing, social engineering, and inadvertent security breaches.
Supply Chain Vulnerabilities:
- Challenge: Dependencies on third-party vendors and suppliers can introduce additional security risks.
- Impact: Compromised security measures from partners can lead to broader network vulnerabilities.
Mitigation Strategies:
Comprehensive Security Framework:
- Strategy: Implement a multi-layered cybersecurity framework that includes firewalls, intrusion detection systems (IDS), and encryption.
- Benefit: Provides robust protection against a variety of cyber threats, ensuring data integrity and system availability.
Regular Security Audits and Assessments:
- Strategy: Conduct periodic security audits and vulnerability assessments to identify and address potential weaknesses.
- Benefit: Proactively detects and mitigates security gaps before they can be exploited by attackers.
Employee Training and Awareness Programs:
- Strategy: Develop ongoing training programs to educate employees about cybersecurity best practices and emerging threats.
- Benefit: Reduces the risk of human error and enhances the organization’s overall security posture.
Secure Integration of Legacy Systems:
- Strategy: Apply security patches, update software, and implement network segmentation to protect legacy systems.
- Benefit: Minimizes vulnerabilities associated with outdated technologies, ensuring secure interoperability with new systems.
Data Encryption and Access Controls:
- Strategy: Encrypt sensitive data both at rest and in transit, and enforce strict access controls based on the principle of least privilege.
- Benefit: Protects data from unauthorized access and ensures that only authorized personnel can access critical information.
Incident Response and Recovery Plans:
- Strategy: Develop and regularly update incident response plans to swiftly address and recover from security breaches.
- Benefit: Minimizes the impact of cyber incidents and ensures rapid restoration of normal operations.
Collaborative Security with Supply Chain Partners:
- Strategy: Establish cybersecurity standards and protocols with suppliers and vendors, and regularly assess their security practices.
- Benefit: Enhances the overall security of the extended supply chain, reducing the risk of external vulnerabilities.
Implementation of Zero Trust Architecture:
- Strategy: Adopt a Zero Trust security model that assumes no entity, inside or outside the network, is inherently trustworthy.
- Benefit: Enhances security by continuously verifying and validating all access requests, reducing the risk of unauthorized access.
Case Example: A leading automotive manufacturer integrated IoT sensors across its production lines for real-time monitoring. To mitigate cybersecurity risks, they implemented a Zero Trust architecture, segmented their network to isolate critical systems, and provided comprehensive cybersecurity training to employees. Additionally, they collaborated closely with their suppliers to ensure that all third-party systems adhered to stringent security standards. As a result, the manufacturer significantly reduced its vulnerability to cyberattacks, ensuring uninterrupted production and safeguarding sensitive data.
Conclusion: Cybersecurity is a critical concern in the era of Industry 4.0, where increased connectivity and data integration elevate the risk of cyber threats. By adopting comprehensive security measures, fostering a culture of cybersecurity awareness, and ensuring robust protection across all layers of the manufacturing ecosystem, manufacturers can effectively mitigate these risks and fully leverage the benefits of Smart Manufacturing.
2. How does the implementation of Digital Twin technology enhance predictive maintenance in manufacturing?
Answer:
Digital Twin Technology in Manufacturing:
A Digital Twin is a virtual replica of a physical asset, system, or process that mirrors its real-time performance, behavior, and condition. In manufacturing, Digital Twin technology leverages data from sensors, IoT devices, and historical records to create a dynamic, interactive model of machinery, production lines, or entire factories.
Enhancement of Predictive Maintenance through Digital Twins:
Real-Time Monitoring and Simulation:
- Function: Digital Twins continuously receive data from their physical counterparts, enabling real-time monitoring of equipment performance and operational conditions.
- Enhancement: By simulating the physical asset’s behavior under various conditions, Digital Twins can predict potential failures before they occur, allowing for timely maintenance interventions.
Data Integration and Analysis:
- Function: Digital Twins integrate data from multiple sources, including sensors, machine logs, and environmental factors.
- Enhancement: Advanced analytics and machine learning algorithms analyze this comprehensive data to identify patterns and anomalies indicative of impending equipment issues.
Condition-Based Maintenance:
- Function: Digital Twins assess the actual condition of equipment rather than relying on predefined maintenance schedules.
- Enhancement: Maintenance activities are triggered based on real-time data and predictive insights, optimizing maintenance resources and reducing unnecessary downtime.
Root Cause Analysis:
- Function: When a potential failure is detected, Digital Twins can simulate different scenarios to determine the root cause.
- Enhancement: This detailed analysis enables more effective and targeted corrective actions, preventing recurring issues and enhancing overall equipment reliability.
Lifecycle Management:
- Function: Digital Twins track the entire lifecycle of machinery, from installation to decommissioning.
- Enhancement: By understanding wear and tear patterns over time, manufacturers can better plan for replacements, upgrades, and maintenance, extending the asset’s useful life and optimizing capital investments.
Optimized Maintenance Scheduling:
- Function: Digital Twins provide insights into the optimal timing for maintenance activities based on equipment usage and condition.
- Enhancement: This optimization minimizes production disruptions, aligns maintenance with production schedules, and maximizes operational efficiency.
Enhanced Collaboration and Decision-Making:
- Function: Digital Twins provide a shared platform for engineers, maintenance teams, and management to collaborate and make informed decisions.
- Enhancement: Facilitates better communication, faster problem-solving, and more strategic maintenance planning, leading to improved operational performance.
Benefits of Using Digital Twins for Predictive Maintenance:
- Reduced Downtime: By predicting and addressing equipment failures before they occur, Digital Twins minimize unplanned downtime and ensure continuous production.
- Cost Savings: Optimizing maintenance schedules and preventing major breakdowns lowers maintenance costs and reduces the need for expensive emergency repairs.
- Extended Equipment Lifespan: Regular, condition-based maintenance helps in preserving machinery, extending its operational life, and maximizing return on investment.
- Improved Safety: Predictive maintenance reduces the risk of catastrophic equipment failures, enhancing workplace safety.
- Enhanced Efficiency: Streamlined maintenance processes and reduced downtime contribute to overall operational efficiency and productivity.
- Data-Driven Insights: Continuous data collection and analysis provide valuable insights into equipment performance, supporting continuous improvement initiatives.
Case Example: A leading aerospace manufacturer implemented Digital Twin technology for its critical assembly machines. By creating virtual replicas of these machines, the company was able to monitor their performance in real-time and simulate various operational scenarios. The Digital Twins analyzed data from vibration sensors, temperature gauges, and usage logs to predict when components would likely fail. This predictive maintenance approach enabled the manufacturer to perform maintenance activities proactively, reducing machine downtime by 35%, cutting maintenance costs by 20%, and extending the lifespan of key machinery components.
Conclusion: Digital Twin technology significantly enhances predictive maintenance in manufacturing by providing real-time insights, enabling condition-based maintenance, and facilitating data-driven decision-making. By accurately predicting equipment failures and optimizing maintenance schedules, Digital Twins contribute to increased operational efficiency, reduced costs, and improved equipment reliability, thereby driving the success of Smart Manufacturing initiatives within the Industry 4.0 framework.
3. In what ways does blockchain technology contribute to transparency and traceability in smart manufacturing environments?
Answer:
Blockchain Technology in Smart Manufacturing:
Blockchain is a decentralized, immutable ledger technology that securely records transactions across multiple computers. In smart manufacturing, blockchain enhances transparency and traceability by providing a secure and tamper-proof record of all activities within the manufacturing process.
Contributions to Transparency and Traceability:
Immutable Record-Keeping:
- Contribution: Blockchain ensures that once data is recorded, it cannot be altered or deleted.
- Impact: Provides a trustworthy and verifiable history of all transactions and activities, ensuring data integrity and preventing fraud or tampering.
End-to-End Traceability:
- Contribution: Records every step of the supply chain, from raw material sourcing to final product delivery.
- Impact: Enables manufacturers and customers to trace the origin and journey of products, ensuring compliance with quality standards and ethical sourcing practices.
Enhanced Supply Chain Visibility:
- Contribution: Shares real-time information across all stakeholders in the supply chain through a shared blockchain ledger.
- Impact: Improves coordination, reduces delays, and enhances the ability to respond to disruptions by providing a clear and comprehensive view of the entire supply chain.
Smart Contracts:
- Contribution: Automates and enforces contractual agreements through self-executing smart contracts on the blockchain.
- Impact: Ensures that transactions and agreements are executed accurately and transparently, reducing the need for intermediaries and minimizing the risk of disputes.
Authenticity Verification:
- Contribution: Verifies the authenticity of components and products by recording unique identifiers on the blockchain.
- Impact: Prevents counterfeiting and ensures that customers receive genuine products, enhancing brand reputation and customer trust.
Regulatory Compliance:
- Contribution: Provides an auditable trail of all manufacturing activities, ensuring compliance with industry regulations and standards.
- Impact: Simplifies the audit process, reduces compliance costs, and ensures that products meet all necessary legal and quality requirements.
Data Security and Privacy:
- Contribution: Protects sensitive manufacturing data through cryptographic encryption and decentralized storage.
- Impact: Ensures that only authorized parties can access and view data, enhancing data security and privacy while maintaining transparency.
Reduction of Manual Errors:
- Contribution: Automates data recording and sharing processes, reducing reliance on manual entry.
- Impact: Minimizes human errors, ensuring that data is accurate and reliable, which is critical for effective traceability and transparency.
Benefits of Blockchain for Transparency and Traceability:
- Increased Trust: Builds trust among stakeholders by providing a transparent and verifiable record of all transactions and processes.
- Enhanced Accountability: Assigns clear ownership and responsibility for each step in the manufacturing process, promoting accountability.
- Improved Efficiency: Streamlines supply chain operations by reducing the need for intermediaries and automating processes through smart contracts.
- Greater Customer Confidence: Enables customers to verify the authenticity and origin of products, enhancing their confidence in the brand.
- Fraud Prevention: Prevents unauthorized alterations and counterfeiting by ensuring data integrity and authenticity.
- Simplified Audits: Facilitates easier and more efficient auditing processes by providing a clear and immutable record of all activities.
Case Example: A global electronics manufacturer implemented blockchain technology to enhance traceability in its supply chain. By recording every transaction and movement of components on the blockchain, the company ensured that each part could be traced back to its origin. This implementation enabled the company to quickly identify and address issues such as defective batches, ensuring that only compliant and high-quality components were used in production. Additionally, customers could verify the authenticity of their products through blockchain records, boosting their trust and satisfaction.
Conclusion: Blockchain technology plays a pivotal role in enhancing transparency and traceability in smart manufacturing environments. By providing an immutable and decentralized ledger for all manufacturing activities, blockchain ensures data integrity, improves supply chain visibility, and fosters trust among stakeholders. These capabilities not only streamline operations and reduce costs but also elevate product quality and customer satisfaction, making blockchain an invaluable tool in the pursuit of excellence within Industry 4.0.
4. How does edge computing differ from cloud computing in the context of smart manufacturing, and what are the advantages of using edge computing?
Answer:
Edge Computing vs. Cloud Computing in Smart Manufacturing:
Edge Computing: Edge computing refers to the decentralized processing of data near the source of data generation, such as on manufacturing equipment, sensors, or local servers. Instead of sending all data to a centralized cloud for processing, edge computing performs data analysis and decision-making at or near the data source.
Cloud Computing: Cloud computing involves centralized data storage and processing on remote servers accessed via the internet. Data from various sources is transmitted to the cloud, where it is stored, processed, and analyzed before results are sent back to the user or device.
Key Differences:
Aspect | Edge Computing | Cloud Computing |
---|---|---|
Data Processing | Localized, near data source | Centralized, remote data centers |
Latency | Low latency due to proximity | Higher latency due to data transmission |
Bandwidth Usage | Reduces bandwidth by processing data locally | High bandwidth usage as data is transmitted to the cloud |
Reliability | More resilient to internet outages | Dependent on internet connectivity |
Security | Enhanced security as sensitive data is processed locally | Potential vulnerabilities during data transmission |
Scalability | Limited by local hardware resources | Highly scalable with cloud infrastructure |
Real-Time Processing | Facilitates real-time or near-real-time processing | Less suitable for real-time applications due to latency |
Cost | Potentially lower operational costs for real-time applications | Costs associated with data storage and transfer |
Advantages of Using Edge Computing in Smart Manufacturing:
Reduced Latency:
- Advantage: Processing data locally minimizes delays in data transmission.
- Impact: Enables real-time or near-real-time decision-making, essential for applications like automated control systems and predictive maintenance.
Bandwidth Optimization:
- Advantage: By processing and filtering data at the edge, only relevant information is sent to the cloud.
- Impact: Reduces the strain on network bandwidth, lowers data transmission costs, and improves overall network efficiency.
Enhanced Reliability:
- Advantage: Edge computing systems can continue to operate independently of cloud connectivity.
- Impact: Ensures continuous operation and data processing even during internet outages, enhancing system resilience and uptime.
Improved Security and Privacy:
- Advantage: Sensitive data can be processed locally without being transmitted over the internet.
- Impact: Reduces the risk of data breaches and enhances data privacy by keeping critical information within the local network.
Scalability and Flexibility:
- Advantage: Edge devices can be added or upgraded independently to meet specific processing needs.
- Impact: Allows manufacturers to scale their operations incrementally without overhauling the entire infrastructure.
Real-Time Analytics:
- Advantage: Enables immediate analysis and response to data, supporting dynamic manufacturing processes.
- Impact: Enhances operational efficiency, reduces waste, and improves product quality through timely interventions.
Energy Efficiency:
- Advantage: Reduces the need for constant data transmission to the cloud, lowering energy consumption associated with data transfer and processing.
- Impact: Contributes to more sustainable manufacturing practices and reduces operational costs.
Localized Control:
- Advantage: Provides granular control over individual machines and processes.
- Impact: Enhances the ability to fine-tune operations and quickly address localized issues without relying on centralized systems.
Case Example: A large automotive manufacturer implemented edge computing in its assembly lines to monitor and control robotic systems in real-time. By processing sensor data locally, the system could immediately detect and respond to anomalies, such as deviations in component placement or assembly speed. This implementation reduced assembly line downtime by 25%, improved product quality by 15%, and decreased reliance on cloud-based data processing, resulting in cost savings and enhanced operational efficiency.
Conclusion: Edge computing offers significant advantages over cloud computing in smart manufacturing by enabling real-time data processing, reducing latency, optimizing bandwidth usage, and enhancing reliability and security. By leveraging edge computing, manufacturers can achieve greater operational efficiency, improved product quality, and more resilient production systems, making it a critical component of Industry 4.0 strategies.
5. What is the role of Artificial Intelligence (AI) in optimizing supply chain management within Industry 4.0?
Answer:
Artificial Intelligence (AI) in Supply Chain Management:
AI plays a transformative role in optimizing supply chain management within the Industry 4.0 framework by leveraging advanced algorithms, machine learning, and data analytics to enhance efficiency, predictability, and responsiveness across the entire supply chain.
Key Roles of AI in Supply Chain Optimization:
Demand Forecasting:
- Role: AI analyzes historical sales data, market trends, and external factors to predict future demand accurately.
- Optimization: Improves inventory planning, reduces stockouts and overstock situations, and aligns production schedules with market demand.
Inventory Management:
- Role: AI algorithms optimize inventory levels by predicting optimal stock quantities and reorder points.
- Optimization: Minimizes holding costs, reduces excess inventory, and ensures timely availability of materials and products.
Predictive Maintenance for Supply Chain Equipment:
- Role: AI monitors the condition of supply chain equipment, such as conveyor systems and logistics machinery, predicting potential failures.
- Optimization: Prevents unexpected downtimes, ensures continuous operation, and extends the lifespan of critical supply chain assets.
Route Optimization:
- Role: AI analyzes various factors like traffic patterns, weather conditions, and delivery schedules to determine the most efficient delivery routes.
- Optimization: Reduces transportation costs, shortens delivery times, and enhances fuel efficiency, contributing to faster and more cost-effective logistics.
Supplier Selection and Risk Management:
- Role: AI evaluates supplier performance based on historical data, financial stability, and compliance records to select the most reliable partners.
- Optimization: Enhances supplier reliability, reduces risks associated with supplier failures, and ensures a steady supply of high-quality materials.
Automated Procurement Processes:
- Role: AI automates procurement tasks such as order placement, invoice processing, and contract management.
- Optimization: Streamlines procurement operations, reduces manual errors, and accelerates the procurement cycle, enhancing overall supply chain efficiency.
Enhanced Visibility and Transparency:
- Role: AI integrates data from various sources to provide real-time visibility into supply chain operations.
- Optimization: Facilitates informed decision-making, improves coordination among stakeholders, and enables proactive management of supply chain disruptions.
Risk Prediction and Mitigation:
- Role: AI identifies potential risks in the supply chain, such as geopolitical issues, natural disasters, or supplier insolvency.
- Optimization: Enables proactive risk mitigation strategies, ensuring supply chain resilience and continuity during disruptions.
Cost Optimization:
- Role: AI analyzes cost structures across the supply chain, identifying areas for cost reduction without compromising quality or service levels.
- Optimization: Lowers overall supply chain costs, enhances profitability, and improves competitive positioning.
Personalization and Customer Service:
- Role: AI tailors supply chain operations to meet specific customer preferences and requirements, such as customized delivery schedules.
- Optimization: Enhances customer satisfaction, loyalty, and retention by providing personalized and responsive supply chain services.
Benefits of AI in Supply Chain Optimization:
- Increased Efficiency: Automates and optimizes various supply chain processes, reducing manual intervention and operational delays.
- Enhanced Accuracy: Improves the precision of demand forecasts, inventory levels, and procurement decisions, minimizing errors and enhancing reliability.
- Cost Savings: Identifies and eliminates inefficiencies, reduces transportation and inventory costs, and optimizes resource utilization.
- Improved Responsiveness: Enables swift adaptation to changing market conditions and customer demands, enhancing supply chain agility.
- Risk Reduction: Anticipates and mitigates potential risks, ensuring supply chain stability and resilience.
- Data-Driven Insights: Provides actionable insights through advanced analytics, supporting strategic decision-making and continuous improvement.
Case Example: A global consumer goods company implemented AI-driven demand forecasting to predict regional sales trends accurately. By analyzing diverse data sources, including social media trends, economic indicators, and historical sales data, the AI system provided highly accurate forecasts. This implementation enabled the company to optimize inventory levels, reduce excess stock by 20%, decrease stockouts by 15%, and improve customer satisfaction through better product availability. Additionally, AI-powered route optimization reduced transportation costs by 10% and improved delivery times, enhancing overall supply chain performance.
Conclusion: Artificial Intelligence is a pivotal enabler of supply chain optimization within Industry 4.0, offering advanced capabilities that enhance forecasting accuracy, operational efficiency, risk management, and cost-effectiveness. By integrating AI into supply chain management, manufacturers can achieve greater agility, resilience, and competitiveness, ensuring that their supply chains are robust and responsive in a rapidly evolving market landscape.
6. How does the integration of Augmented Reality (AR) and Virtual Reality (VR) technologies improve training and maintenance processes in smart manufacturing?
Answer:
Augmented Reality (AR) and Virtual Reality (VR) in Smart Manufacturing:
AR and VR technologies are increasingly being integrated into smart manufacturing environments to enhance training, maintenance, and operational efficiency. These immersive technologies provide interactive and realistic experiences that facilitate better learning, problem-solving, and decision-making.
Improvements in Training Processes:
Interactive Training Modules:
- Improvement: AR and VR create immersive training simulations that replicate real-world manufacturing scenarios.
- Benefit: Provides hands-on experience without the risks associated with actual machinery, enhancing learning outcomes and retention rates.
Remote Training Capabilities:
- Improvement: VR-based training can be accessed remotely, allowing employees from different locations to participate in the same training program.
- Benefit: Reduces the need for physical training facilities, lowers training costs, and ensures consistent training quality across the organization.
Customized Learning Experiences:
- Improvement: AR and VR can tailor training programs to individual learning paces and styles, offering personalized instruction.
- Benefit: Enhances the effectiveness of training by addressing the specific needs and preferences of each learner, leading to better skill acquisition.
Real-Time Feedback and Assessment:
- Improvement: These technologies can provide instant feedback on performance during training exercises.
- Benefit: Helps trainees quickly identify and correct mistakes, accelerating the learning process and improving competency levels.
Safe Environment for Skill Development:
- Improvement: VR simulations allow employees to practice operating complex or dangerous equipment in a controlled, virtual environment.
- Benefit: Enhances safety by enabling skill development without exposing trainees to actual hazards, reducing the likelihood of accidents during real operations.
Improvements in Maintenance Processes:
Guided Maintenance Procedures:
- Improvement: AR overlays provide step-by-step instructions and visual cues directly onto machinery during maintenance tasks.
- Benefit: Increases the accuracy and efficiency of maintenance activities, reducing the time required to perform repairs and minimizing errors.
Remote Assistance and Collaboration:
- Improvement: AR enables remote experts to guide on-site technicians through complex maintenance procedures in real-time.
- Benefit: Enhances problem-solving capabilities, reduces downtime by speeding up repairs, and leverages expertise without requiring physical presence.
Virtual Maintenance Simulations:
- Improvement: VR simulations allow maintenance teams to practice procedures and troubleshoot issues in a virtual replica of the machinery.
- Benefit: Prepares technicians for real-world scenarios, improving their readiness and effectiveness when dealing with actual equipment issues.
Predictive Maintenance Insights:
- Improvement: AR can display real-time data and predictive analytics related to equipment health during maintenance tasks.
- Benefit: Enables more informed decision-making, allowing technicians to address potential issues proactively and extend the lifespan of machinery.
Documentation and Knowledge Sharing:
- Improvement: AR can link to digital manuals, schematics, and instructional videos, providing easy access to critical information during maintenance.
- Benefit: Streamlines the maintenance process, ensures consistency in procedures, and facilitates knowledge sharing across the organization.
Benefits of AR and VR Integration:
- Enhanced Learning and Skill Acquisition: Immersive training experiences lead to better understanding and retention of complex concepts and procedures.
- Increased Operational Efficiency: Guided and informed maintenance processes reduce downtime and improve equipment reliability.
- Cost Savings: Virtual training reduces the need for physical training materials and facilities, while efficient maintenance minimizes repair costs and production losses.
- Improved Safety: Safe training and maintenance environments prevent accidents and injuries, fostering a safer workplace.
- Scalability and Flexibility: AR and VR training programs can be easily scaled and updated to accommodate new technologies and processes, ensuring that the workforce remains proficient and adaptable.
Case Example: A leading electronics manufacturer integrated AR into its maintenance operations by equipping technicians with AR headsets. These headsets provided real-time, step-by-step maintenance instructions overlaid onto the machinery. When a technician encountered an issue with a complex assembly machine, the AR system guided them through the diagnostic and repair process, highlighting critical components and tools needed. This implementation reduced maintenance time by 30%, decreased error rates, and enhanced the overall effectiveness of the maintenance team. Additionally, the company utilized VR for training new employees, allowing them to practice operating and maintaining machinery in a risk-free virtual environment, resulting in faster onboarding and higher competency levels.
Conclusion: The integration of Augmented Reality and Virtual Reality technologies in smart manufacturing significantly improves training and maintenance processes by providing immersive, interactive, and efficient solutions. These technologies enhance learning outcomes, increase operational efficiency, reduce costs, and promote a safer work environment. By leveraging AR and VR, manufacturers can ensure that their workforce is well-trained, skilled, and capable of maintaining high levels of productivity and quality in an increasingly complex and automated manufacturing landscape.
7. How does the use of Robotics Process Automation (RPA) enhance operational efficiency in smart manufacturing?
Answer:
Robotic Process Automation (RPA) in Smart Manufacturing:
Robotic Process Automation (RPA) involves using software robots or “bots” to automate repetitive, rule-based tasks typically performed by humans. In smart manufacturing, RPA enhances operational efficiency by streamlining processes, reducing errors, and freeing up human resources for more strategic activities.
Enhancements to Operational Efficiency through RPA:
Automating Repetitive Tasks:
- Enhancement: RPA automates routine and monotonous tasks such as data entry, order processing, and inventory management.
- Impact: Increases speed and accuracy of operations, reduces human error, and allows employees to focus on higher-value tasks like problem-solving and innovation.
Streamlining Supply Chain Operations:
- Enhancement: RPA manages tasks such as order tracking, shipment scheduling, and supplier communications.
- Impact: Enhances supply chain visibility, improves coordination, and ensures timely delivery of materials and products, reducing lead times and operational delays.
Improving Data Management and Reporting:
- Enhancement: RPA collects, processes, and analyzes data from various sources, generating real-time reports and dashboards.
- Impact: Provides actionable insights for decision-making, enhances data accuracy, and supports continuous monitoring of key performance indicators (KPIs).
Enhancing Quality Control:
- Enhancement: RPA automates quality inspection processes, such as collecting inspection data, comparing it against standards, and flagging defects.
- Impact: Ensures consistent quality control, reduces inspection time, and minimizes the risk of human oversight, leading to higher product quality and customer satisfaction.
Optimizing Inventory Management:
- Enhancement: RPA tracks inventory levels, automates reordering processes, and manages stock movements.
- Impact: Prevents stockouts and overstock situations, optimizes inventory turnover, and reduces holding costs, improving overall inventory efficiency.
Facilitating Compliance and Documentation:
- Enhancement: RPA ensures that all manufacturing processes adhere to regulatory standards by automating compliance checks and maintaining accurate documentation.
- Impact: Simplifies compliance management, reduces the risk of non-compliance penalties, and ensures that all necessary records are up-to-date and easily accessible.
Enhancing Maintenance Processes:
- Enhancement: RPA automates the scheduling of maintenance activities, tracks maintenance logs, and monitors equipment performance data.
- Impact: Ensures timely maintenance, extends equipment lifespan, and reduces the likelihood of unexpected breakdowns, thereby enhancing overall operational reliability.
Cost Reduction:
- Enhancement: By automating labor-intensive tasks, RPA reduces the need for manual labor and lowers operational costs.
- Impact: Increases profitability by minimizing labor expenses and reducing costs associated with errors and inefficiencies.
Scalability and Flexibility:
- Enhancement: RPA systems can be easily scaled up or down based on production demands and operational needs.
- Impact: Provides manufacturers with the flexibility to adapt to market fluctuations and scale operations without significant additional investments.
Enhanced Customer Service:
- Enhancement: RPA manages customer inquiries, processes orders, and handles returns efficiently.
- Impact: Improves response times, enhances customer satisfaction, and ensures a seamless customer experience, fostering loyalty and repeat business.
Benefits of RPA in Smart Manufacturing:
- Increased Productivity: Automates time-consuming tasks, allowing human workers to concentrate on more strategic and creative endeavors.
- Improved Accuracy: Reduces the incidence of errors in repetitive tasks, ensuring higher data integrity and process reliability.
- Faster Operations: Accelerates process execution, leading to shorter cycle times and quicker turnaround on orders and services.
- Enhanced Compliance: Maintains consistent adherence to regulatory standards through automated compliance checks and documentation.
- Cost Efficiency: Lowers operational costs by reducing the need for manual labor and minimizing errors that can lead to financial losses.
- Greater Scalability: Easily adapts to increased production demands without the need for proportional increases in workforce or resources.
- Enhanced Employee Satisfaction: Frees employees from mundane tasks, increasing job satisfaction and reducing turnover rates by allowing them to engage in more meaningful work.
Case Example: A large manufacturing company implemented RPA to automate its inventory management and order processing systems. By deploying software bots to handle tasks such as updating inventory levels, generating purchase orders, and processing customer orders, the company achieved a 40% reduction in order processing time and a 25% decrease in inventory holding costs. Additionally, the automation of these processes reduced human errors by 30%, enhancing overall operational accuracy and efficiency.
Conclusion: Robotic Process Automation significantly enhances operational efficiency in smart manufacturing by automating repetitive tasks, improving data accuracy, streamlining supply chain operations, and reducing costs. By leveraging RPA, manufacturers can achieve higher productivity, better quality control, and greater flexibility, positioning themselves for sustained success in the competitive landscape of Industry 4.0.
8. What is the significance of Digital Twin technology in enhancing product lifecycle management (PLM) within Industry 4.0?
Answer:
Digital Twin Technology in Product Lifecycle Management (PLM):
A Digital Twin is a virtual replica of a physical product, process, or system that simulates its real-time performance, behavior, and lifecycle. In the context of Product Lifecycle Management (PLM) within Industry 4.0, Digital Twin technology plays a crucial role in enhancing the management and optimization of products from conception through design, manufacturing, and maintenance to end-of-life disposal.
Significance of Digital Twin in Enhancing PLM:
Enhanced Design and Development:
- Significance: Digital Twins enable detailed simulation and testing of product designs before physical prototypes are built.
- Enhancement: Facilitates rapid iteration and optimization of designs, reducing development time and costs while ensuring higher product quality and performance.
Real-Time Monitoring and Feedback:
- Significance: During the manufacturing phase, Digital Twins monitor real-time data from production processes.
- Enhancement: Provides immediate feedback on manufacturing performance, allowing for real-time adjustments to optimize production efficiency and quality.
Predictive Maintenance and Reliability:
- Significance: Digital Twins simulate the operational behavior and wear of products in use.
- Enhancement: Predicts maintenance needs and potential failures, enabling proactive maintenance scheduling that extends product lifespan and reduces downtime.
Lifecycle Optimization:
- Significance: Digital Twins provide a comprehensive view of the entire product lifecycle, integrating data from various stages.
- Enhancement: Identifies opportunities for improvement at each stage, from design to disposal, ensuring that the product remains efficient, cost-effective, and environmentally friendly throughout its lifecycle.
Improved Collaboration and Communication:
- Significance: Digital Twins serve as a centralized digital representation that can be accessed and shared by different stakeholders, including designers, engineers, manufacturers, and service teams.
- Enhancement: Enhances collaboration by providing a common reference point, ensuring that all teams are aligned and can work together seamlessly to address issues and implement improvements.
Customization and Personalization:
- Significance: Digital Twins can simulate and manage customized product configurations.
- Enhancement: Facilitates mass customization by enabling the efficient management of multiple product variants without compromising on quality or production efficiency.
Data-Driven Decision Making:
- Significance: Digital Twins collect and analyze vast amounts of data throughout the product lifecycle.
- Enhancement: Empowers manufacturers to make informed, data-driven decisions that enhance product design, manufacturing processes, and post-sales support.
Sustainability and Environmental Impact:
- Significance: Digital Twins can simulate the environmental impact of products, including energy consumption and material usage.
- Enhancement: Helps in designing more sustainable products and optimizing manufacturing processes to reduce environmental footprints, supporting corporate sustainability goals.
End-of-Life Management:
- Significance: Digital Twins facilitate the planning and execution of product recycling, remanufacturing, or disposal.
- Enhancement: Ensures that products are managed responsibly at the end of their lifecycle, promoting circular economy practices and compliance with environmental regulations.
Benefits of Digital Twin Integration in PLM:
- Reduced Time-to-Market: Accelerates product development cycles through efficient design validation and optimization.
- Cost Savings: Lowers development and maintenance costs by identifying and addressing issues early in the lifecycle.
- Enhanced Product Quality: Improves reliability and performance by enabling continuous monitoring and optimization.
- Increased Agility: Allows manufacturers to quickly adapt to market changes and customer demands through flexible and data-driven PLM processes.
- Sustainability: Promotes eco-friendly product design and manufacturing practices, contributing to environmental stewardship.
- Competitive Advantage: Provides insights and capabilities that differentiate products and manufacturing processes, enhancing market competitiveness.
Case Example: A leading aerospace company implemented Digital Twin technology to manage the lifecycle of its aircraft engines. By creating a virtual replica of each engine, the company could monitor performance in real-time, predict maintenance needs, and optimize operational parameters. This implementation resulted in a 20% reduction in maintenance costs, a 15% increase in engine reliability, and an extended operational lifespan of the engines. Additionally, the Digital Twins facilitated better collaboration between design, manufacturing, and maintenance teams, leading to continuous improvements in engine design and performance.
Conclusion: Digital Twin technology is a pivotal element in enhancing Product Lifecycle Management within Industry 4.0. By providing a comprehensive, real-time digital representation of products, Digital Twins enable manufacturers to optimize design, manufacturing, maintenance, and end-of-life processes. This integration leads to improved product quality, reduced costs, enhanced collaboration, and greater sustainability, ultimately driving the success and competitiveness of manufacturers in the modern industrial landscape.
9. How does the concept of Smart Factory integrate various Industry 4.0 technologies to create a highly automated and efficient manufacturing environment?
Answer:
Smart Factory Concept in Industry 4.0:
A Smart Factory embodies the principles of Industry 4.0 by integrating advanced technologies to create a highly automated, interconnected, and efficient manufacturing environment. It leverages technologies such as the Internet of Things (IoT), artificial intelligence (AI), robotics, big data analytics, cloud computing, and augmented reality (AR) to optimize production processes, enhance flexibility, and improve overall operational performance.
Integration of Industry 4.0 Technologies in Smart Factories:
Internet of Things (IoT):
- Integration: IoT devices and sensors are deployed across the factory floor to collect real-time data from machines, equipment, and production processes.
- Outcome: Enables continuous monitoring, data-driven decision-making, and proactive maintenance, enhancing operational efficiency and reducing downtime.
Artificial Intelligence (AI) and Machine Learning:
- Integration: AI algorithms analyze data collected from IoT devices to identify patterns, predict outcomes, and optimize processes.
- Outcome: Facilitates predictive maintenance, quality control, demand forecasting, and process optimization, leading to increased productivity and reduced operational costs.
Robotics and Automation:
- Integration: Advanced robots and automated systems handle repetitive, hazardous, or complex tasks with high precision and speed.
- Outcome: Enhances production speed, accuracy, and consistency while reducing labor costs and minimizing human error.
Big Data Analytics:
- Integration: Big data platforms aggregate and analyze vast amounts of data generated by IoT devices, machines, and business systems.
- Outcome: Provides actionable insights for process improvements, resource optimization, and strategic decision-making, driving continuous operational enhancement.
Cloud Computing:
- Integration: Cloud platforms store and process data from various sources, enabling scalable and flexible data management.
- Outcome: Supports real-time data access, collaboration across different locations, and seamless integration of new technologies, enhancing overall factory agility and responsiveness.
Digital Twin Technology:
- Integration: Digital Twins create virtual replicas of physical assets and processes, simulating their behavior under different conditions.
- Outcome: Enables virtual testing, optimization, and predictive maintenance, reducing the need for physical prototypes and minimizing risks during production changes.
Augmented Reality (AR) and Virtual Reality (VR):
- Integration: AR and VR tools assist in training, maintenance, and design processes by providing immersive and interactive experiences.
- Outcome: Enhances worker training, facilitates remote maintenance support, and improves the accuracy of design and assembly processes, leading to higher productivity and reduced errors.
Additive Manufacturing (3D Printing):
- Integration: 3D printing technology enables on-demand production of complex components and prototypes.
- Outcome: Reduces lead times, allows for greater customization, and minimizes material waste, enhancing the factory’s ability to respond quickly to market demands.
Cybersecurity Solutions:
- Integration: Robust cybersecurity measures protect the interconnected systems and data within the Smart Factory from cyber threats.
- Outcome: Ensures data integrity, protects intellectual property, and maintains operational continuity by preventing unauthorized access and cyberattacks.
Edge Computing:
- Integration: Edge computing processes data locally at the source, reducing the reliance on centralized cloud servers.
- Outcome: Minimizes latency, enhances real-time decision-making, and improves the reliability of critical manufacturing processes by enabling immediate data processing and response.
Characteristics of a Smart Factory:
- Interconnectivity: Seamless communication between machines, systems, and humans through integrated networks.
- Autonomy: Systems can operate independently, making decisions based on real-time data without human intervention.
- Adaptability: Ability to quickly adjust to changes in production demands, product variations, and market conditions.
- Data-Driven: Utilizes data analytics to inform and optimize every aspect of the manufacturing process.
- Sustainability: Implements energy-efficient practices, waste reduction, and sustainable resource management to minimize environmental impact.
Benefits of Smart Factory Integration:
- Increased Efficiency: Streamlines operations through automation and real-time process optimization, leading to higher productivity and reduced operational costs.
- Enhanced Quality: Maintains consistent product quality through precise control and continuous monitoring of production processes.
- Greater Flexibility: Quickly adapts to changes in demand, product designs, and market conditions, enabling rapid response and customization.
- Improved Decision-Making: Leverages data-driven insights to make informed and strategic decisions, enhancing overall operational performance.
- Reduced Downtime: Predictive maintenance and real-time monitoring minimize equipment failures and unplanned downtime, ensuring continuous production.
- Cost Savings: Optimizes resource utilization, reduces waste, and lowers maintenance and operational costs through efficient process management.
- Enhanced Worker Productivity: Automates mundane tasks, allowing human workers to focus on more complex and value-added activities, increasing overall workforce productivity.
- Sustainability: Implements sustainable manufacturing practices, reducing environmental impact and promoting corporate social responsibility.
Case Example: A leading automotive manufacturer transformed its production facility into a Smart Factory by integrating IoT sensors, AI-driven analytics, robotic automation, and Digital Twin technology. IoT sensors monitored machine performance and environmental conditions in real-time, while AI algorithms analyzed the data to predict maintenance needs and optimize production schedules. Robotic arms handled assembly tasks with high precision, and Digital Twins simulated production scenarios to identify and implement process improvements. This comprehensive integration resulted in a 30% increase in production efficiency, a 20% reduction in maintenance costs, and a significant improvement in product quality, demonstrating the transformative impact of a Smart Factory within the Industry 4.0 framework.
Conclusion: The Smart Factory concept epitomizes the convergence of various Industry 4.0 technologies to create a highly automated, efficient, and adaptable manufacturing environment. By integrating IoT, AI, robotics, big data analytics, cloud computing, and other advanced technologies, Smart Factories achieve unprecedented levels of operational excellence, quality, and flexibility. This holistic approach not only enhances productivity and reduces costs but also positions manufacturers to thrive in the dynamic and competitive landscape of modern manufacturing.
10. What ethical considerations must manufacturers address when implementing AI and automation in their production processes?
Answer:
Ethical Considerations in Implementing AI and Automation:
As manufacturers integrate Artificial Intelligence (AI) and automation into their production processes, several ethical considerations emerge that must be thoughtfully addressed to ensure responsible and sustainable practices.
Impact on Employment:
- Consideration: Automation can lead to job displacement as machines and AI systems take over tasks previously performed by humans.
- Ethical Concern: Potential unemployment and loss of livelihoods for workers.
- Mitigation Strategies:
- Invest in reskilling and upskilling programs to transition employees to new roles.
- Promote human-machine collaboration, where AI and robots augment rather than replace human workers.
- Develop new job opportunities in areas such as AI maintenance, programming, and oversight.
Bias and Fairness in AI Algorithms:
- Consideration: AI systems can inadvertently perpetuate biases present in training data or algorithm design.
- Ethical Concern: Discrimination against certain groups of workers or customers, leading to unfair treatment and inequality.
- Mitigation Strategies:
- Ensure diverse and representative datasets for training AI models.
- Implement regular audits and testing to identify and eliminate biases.
- Incorporate fairness and transparency principles in AI development and deployment.
Data Privacy and Security:
- Consideration: AI and automation systems collect and process vast amounts of data, including personal and sensitive information.
- Ethical Concern: Risks of data breaches, unauthorized access, and misuse of personal data.
- Mitigation Strategies:
- Implement robust data encryption and security measures.
- Adhere to data protection regulations and best practices.
- Ensure transparency in data collection, usage, and storage policies.
Transparency and Explainability:
- Consideration: AI decision-making processes can be complex and opaque, making it difficult to understand how conclusions are reached.
- Ethical Concern: Lack of accountability and trust in AI systems, especially in critical decision-making scenarios.
- Mitigation Strategies:
- Develop AI models with explainable and interpretable outputs.
- Provide clear documentation and rationale for AI-driven decisions.
- Enable human oversight and intervention in AI processes.
Accountability and Responsibility:
- Consideration: Determining who is accountable for decisions made by AI and automated systems can be challenging.
- Ethical Concern: Unclear lines of responsibility in cases of errors, accidents, or malfunctions.
- Mitigation Strategies:
- Establish clear policies outlining accountability for AI and automation outcomes.
- Implement governance structures that oversee AI deployment and ethical compliance.
- Ensure that human operators remain responsible for critical decisions and system oversight.
Environmental Impact:
- Consideration: The production and operation of AI and automation technologies can have significant environmental footprints.
- Ethical Concern: Resource consumption, energy usage, and electronic waste contribute to environmental degradation.
- Mitigation Strategies:
- Adopt sustainable practices in the manufacturing and disposal of AI and automation equipment.
- Optimize energy efficiency in AI and robotic systems.
- Promote the recycling and responsible disposal of electronic components.
Human Dignity and Well-Being:
- Consideration: The introduction of AI and automation can affect the psychological and social well-being of workers.
- Ethical Concern: Stress, loss of job satisfaction, and diminished human interaction in the workplace.
- Mitigation Strategies:
- Foster a positive work environment that values human contributions and promotes well-being.
- Ensure that automation enhances rather than diminishes human roles and engagement.
- Provide support systems for employees affected by automation, including counseling and career guidance.
Intellectual Property and Ownership:
- Consideration: AI-generated innovations and automated processes can complicate intellectual property (IP) rights.
- Ethical Concern: Unclear ownership of AI-generated works and processes, leading to potential IP disputes.
- Mitigation Strategies:
- Define clear IP policies that address AI-generated content and inventions.
- Establish legal frameworks that recognize and protect the rights of stakeholders in AI-driven innovation.
Benefits of Addressing Ethical Considerations:
- Trust and Reputation: Ethical practices build trust with employees, customers, and stakeholders, enhancing the organization’s reputation.
- Sustainable Growth: Responsible implementation of AI and automation supports long-term sustainability and reduces the risk of legal and ethical conflicts.
- Employee Engagement: Valuing and supporting workers through ethical practices fosters a motivated and loyal workforce.
- Innovation and Competitiveness: Ethical AI practices encourage responsible innovation, maintaining competitiveness while adhering to societal values.
Case Example: A major electronics manufacturer introduced AI-powered quality inspection systems to enhance product consistency. To address ethical concerns, the company ensured that the AI models were trained on diverse and unbiased datasets to prevent discrimination in defect detection. They also implemented transparent reporting mechanisms, allowing human inspectors to review and override AI decisions when necessary. Additionally, the company invested in reskilling programs for employees affected by the automation, enabling them to transition to roles focused on AI system maintenance and oversight. This holistic approach ensured that the integration of AI upheld ethical standards, maintained employee trust, and enhanced overall product quality.
Conclusion: As manufacturers embrace AI and automation within Industry 4.0, addressing ethical considerations is paramount to ensuring responsible and sustainable practices. By proactively tackling issues related to employment, bias, data privacy, transparency, accountability, environmental impact, human well-being, and intellectual property, manufacturers can harness the full potential of AI and automation while maintaining trust, fairness, and integrity in their operations. This ethical approach not only safeguards stakeholders but also fosters a culture of innovation and excellence, driving long-term success in the competitive manufacturing landscape.
11. How can blockchain technology improve supplier relationship management in smart manufacturing?
Answer:
Blockchain Technology in Supplier Relationship Management (SRM):
Blockchain technology offers a decentralized, transparent, and immutable ledger that can revolutionize Supplier Relationship Management (SRM) in smart manufacturing. By integrating blockchain into SRM processes, manufacturers can enhance transparency, trust, efficiency, and collaboration with their suppliers.
Improvements in Supplier Relationship Management through Blockchain:
Enhanced Transparency and Traceability:
- Improvement: Blockchain records every transaction and interaction between manufacturers and suppliers in a transparent and immutable manner.
- Benefit: Enables both parties to trace the origin, movement, and transformation of goods and materials throughout the supply chain, ensuring accountability and authenticity.
Secure and Efficient Data Sharing:
- Improvement: Blockchain facilitates secure and real-time sharing of data between manufacturers and suppliers without the need for intermediaries.
- Benefit: Reduces the risk of data breaches and unauthorized access while streamlining communication and information flow, enhancing operational efficiency.
Automated Smart Contracts:
- Improvement: Smart contracts on the blockchain automatically execute and enforce contractual agreements when predefined conditions are met.
- Benefit: Ensures timely and accurate execution of contracts, reduces administrative overhead, and minimizes disputes related to contract fulfillment.
Improved Supplier Verification and Compliance:
- Improvement: Blockchain provides a verifiable and tamper-proof record of supplier certifications, compliance documents, and performance metrics.
- Benefit: Simplifies the verification process, ensures that suppliers meet regulatory and quality standards, and enhances trust between parties.
Reduction of Fraud and Counterfeiting:
- Improvement: Blockchain’s immutable ledger ensures that all transactions are recorded accurately and cannot be altered retroactively.
- Benefit: Prevents fraudulent activities, such as counterfeit goods entering the supply chain, protecting brand integrity and customer trust.
Optimized Inventory Management:
- Improvement: Real-time visibility into inventory levels and movements through blockchain data allows for better coordination with suppliers.
- Benefit: Enhances inventory accuracy, reduces stockouts and overstock situations, and supports just-in-time (JIT) inventory practices.
Streamlined Payment Processes:
- Improvement: Blockchain facilitates secure and automated payment transactions between manufacturers and suppliers.
- Benefit: Reduces payment delays, lowers transaction costs, and minimizes the risk of payment fraud, ensuring timely financial settlements.
Enhanced Collaboration and Trust:
- Improvement: The transparent and secure nature of blockchain fosters a higher level of trust and collaboration between manufacturers and suppliers.
- Benefit: Promotes long-term partnerships, encourages information sharing, and facilitates joint problem-solving and innovation.
Efficient Dispute Resolution:
- Improvement: Blockchain’s transparent records provide clear evidence of transactions and contract terms, simplifying the resolution of disputes.
- Benefit: Reduces the time and cost associated with resolving conflicts, enhancing overall supplier relationships.
Sustainability and Ethical Sourcing:
- Improvement: Blockchain can track and verify the sustainability and ethical practices of suppliers, such as fair labor standards and environmentally friendly sourcing.
- Benefit: Ensures that manufacturers maintain responsible and sustainable supply chains, aligning with corporate social responsibility (CSR) goals and consumer expectations.
Benefits of Blockchain in SRM:
- Increased Trust: Transparent and immutable records build trust between manufacturers and suppliers, fostering reliable and consistent relationships.
- Operational Efficiency: Automating contract execution and streamlining data sharing reduce manual processes, saving time and resources.
- Risk Mitigation: Enhanced traceability and verification reduce risks related to fraud, counterfeiting, and non-compliance.
- Cost Savings: Lower administrative and transaction costs through automation and reduced reliance on intermediaries.
- Enhanced Decision-Making: Real-time data and insights enable informed decision-making, improving strategic planning and supplier selection.
Case Example: A global automotive manufacturer implemented a blockchain-based SRM system to manage its extensive network of suppliers. By recording all supplier transactions, certifications, and compliance data on the blockchain, the manufacturer achieved full traceability of parts and materials from source to assembly. Smart contracts were used to automate payment processes upon delivery and inspection of goods, ensuring timely settlements. This implementation resulted in a 25% reduction in administrative costs, a 30% decrease in payment processing times, and a significant improvement in supplier trust and collaboration. Additionally, the ability to trace the origin of components enhanced the manufacturer’s ability to ensure quality and compliance, reducing the incidence of defective parts and recalls.
Conclusion: Blockchain technology significantly enhances Supplier Relationship Management in smart manufacturing by providing unparalleled transparency, security, and efficiency. By leveraging blockchain’s capabilities, manufacturers can build more trustworthy and collaborative relationships with suppliers, optimize supply chain operations, and ensure compliance with quality and ethical standards. This integration not only improves operational performance and reduces costs but also strengthens the overall resilience and sustainability of the manufacturing supply chain within the Industry 4.0 ecosystem.
12. What future advancements in Industry 4.0 technologies can further transform smart manufacturing, and how might they impact the industry?
Answer:
Future Advancements in Industry 4.0 Technologies:
As Industry 4.0 continues to evolve, several emerging technologies and advancements are poised to further transform smart manufacturing. These future advancements promise to enhance automation, connectivity, intelligence, and sustainability, driving significant changes in the manufacturing landscape.
1. Advanced Artificial Intelligence and Machine Learning:
- Advancement: Development of more sophisticated AI and machine learning algorithms capable of deeper predictive analytics, autonomous decision-making, and adaptive learning.
- Impact: Enhances the ability to optimize complex manufacturing processes, improve product quality, and enable fully autonomous production systems. AI-driven robots could adapt in real-time to changing conditions without human intervention, increasing efficiency and flexibility.
2. Quantum Computing:
- Advancement: Utilization of quantum computing to solve complex optimization and simulation problems at unprecedented speeds.
- Impact: Revolutionizes areas such as supply chain optimization, material science, and product design by enabling faster and more accurate simulations and optimizations, leading to innovative products and more efficient manufacturing processes.
3. Extended Reality (XR) Integration:
- Advancement: Further integration of augmented reality (AR), virtual reality (VR), and mixed reality (MR) into manufacturing processes.
- Impact: Enhances training, maintenance, and design activities by providing more immersive and interactive experiences. XR can facilitate remote collaboration, advanced prototyping, and real-time visualization of complex processes, improving accuracy and reducing errors.
4. 5G and Beyond Connectivity:
- Advancement: Deployment of 5G networks and future advancements in wireless connectivity.
- Impact: Provides ultra-low latency, high bandwidth, and reliable connectivity, enabling real-time data exchange, remote monitoring, and seamless integration of IoT devices. Enhances the performance of autonomous robots and real-time analytics, leading to more responsive and agile manufacturing environments.
5. Autonomous Supply Chains:
- Advancement: Development of fully autonomous supply chains powered by AI, IoT, blockchain, and robotics.
- Impact: Creates self-managing supply chains that can autonomously handle procurement, production, distribution, and logistics. Increases efficiency, reduces human intervention, and enhances resilience against disruptions.
6. Advanced Robotics and Collaborative Robots (Cobots):
- Advancement: Continued innovation in robotics, including more advanced cobots with enhanced dexterity, sensory capabilities, and AI integration.
- Impact: Enables robots to perform more complex and delicate tasks, work safely alongside humans, and adapt to dynamic production environments. Improves productivity, quality, and worker safety.
7. Sustainable and Green Manufacturing Technologies:
- Advancement: Innovations focused on sustainability, such as carbon capture technologies, renewable energy integration, and eco-friendly materials.
- Impact: Reduces the environmental footprint of manufacturing operations, aligns with global sustainability goals, and meets increasing consumer demand for environmentally responsible products.
8. Digital Thread and Seamless Integration:
- Advancement: Development of a comprehensive digital thread that seamlessly connects all aspects of the product lifecycle from design to disposal.
- Impact: Enhances traceability, quality control, and lifecycle management by ensuring that data flows seamlessly across all stages of production. Facilitates continuous improvement and more informed decision-making.
9. Human-Machine Interfaces (HMIs) Evolution:
- Advancement: Advancement in HMIs, including gesture recognition, voice control, and brain-computer interfaces (BCIs).
- Impact: Improves the ease of interaction between humans and machines, enhancing usability, efficiency, and safety. Enables more intuitive and natural control of manufacturing systems.
10. Bio-Manufacturing and Synthetic Biology: – Advancement: Integration of bio-manufacturing techniques and synthetic biology into traditional manufacturing processes. – Impact: Expands the range of producible materials and products, enabling the creation of bio-based components, sustainable materials, and innovative biomedical devices. Promotes sustainability and opens new avenues for product development.
11. Edge AI and Intelligent Sensors: – Advancement: Development of AI capabilities at the edge, integrated with intelligent sensors and devices. – Impact: Enables real-time, localized data processing and decision-making without relying on centralized cloud servers. Enhances responsiveness, reduces latency, and improves the efficiency of manufacturing operations.
12. Smart Factories with Fully Integrated AI and IoT: – Advancement: Evolution towards fully integrated smart factories where AI, IoT, robotics, and other technologies are seamlessly interconnected. – Impact: Creates highly autonomous and self-optimizing manufacturing environments that can dynamically adjust to changing conditions, optimize resource usage, and continuously improve processes. Enhances overall operational efficiency, flexibility, and competitiveness.
Potential Impacts on the Industry:
- Increased Productivity and Efficiency: Advanced technologies streamline operations, reduce downtime, and optimize resource utilization, leading to higher productivity and lower operational costs.
- Enhanced Product Quality and Customization: AI-driven quality control, precise robotics, and flexible production systems ensure consistent product quality while enabling mass customization.
- Greater Agility and Responsiveness: Smart manufacturing technologies enable rapid adaptation to market changes, customer demands, and supply chain disruptions, enhancing the agility and resilience of manufacturing operations.
- Sustainability and Environmental Responsibility: Innovations focused on sustainability reduce the environmental impact of manufacturing, supporting global sustainability goals and meeting consumer expectations for eco-friendly products.
- Workforce Transformation: Automation and AI redefine workforce roles, requiring new skills and fostering a collaborative environment where humans and machines work together to achieve optimal outcomes.
- Competitive Advantage: Early adopters of advanced Industry 4.0 technologies gain a significant competitive edge through enhanced operational capabilities, innovation, and responsiveness.
- Innovation and New Business Models: Emerging technologies drive the creation of new products, services, and business models, expanding the scope and potential of the manufacturing industry.
Case Example: A leading electronics manufacturer adopted several future Industry 4.0 technologies, including advanced AI algorithms for predictive maintenance, 5G-enabled IoT devices for real-time monitoring, and blockchain for supply chain transparency. Additionally, they integrated augmented reality (AR) for remote maintenance support and developed a comprehensive digital thread connecting design, manufacturing, and service processes. These advancements resulted in a 40% increase in operational efficiency, a 30% reduction in maintenance costs, enhanced product quality, and improved customer satisfaction. The company also achieved significant sustainability goals by optimizing energy usage and reducing material waste through data-driven insights and automation.
Conclusion: Future advancements in Industry 4.0 technologies hold immense potential to further transform smart manufacturing by enhancing automation, connectivity, intelligence, and sustainability. As these technologies continue to evolve, manufacturers that strategically adopt and integrate them will benefit from increased productivity, improved quality, greater agility, and a stronger competitive position. Embracing these innovations will be crucial for manufacturers to thrive in the rapidly changing and increasingly complex landscape of modern manufacturing.
Conclusion:
The above thought-provoking questions and detailed answers delve into the multifaceted aspects of Smart Manufacturing and Industry 4.0. Covering critical topics such as cybersecurity, Digital Twin technology, blockchain integration, edge computing, AI in supply chain management, AR/VR applications, RPA, ethical considerations, supplier relationship management, future technological advancements, and the role of blockchain in transparency, these questions provide a comprehensive exploration of how advanced technologies are reshaping the manufacturing landscape. Engaging with these questions equips students and professionals with the knowledge to understand, implement, and innovate within the framework of Industry 4.0, ensuring they are well-prepared to navigate and contribute to the future of smart manufacturing.